Furthermore, ensuring steady flow in the risers is important to maintaining a high production, reducing flaring, and protecting the downstream equipment, i.e., the separation and cleaning facilities which follow the risers.
Unsteady flow can arise due to slugs in the riser, and they cause large oscillations in the pressure as well as changes in the oil and gas ratio.
In severe cases, the pressure oscillations can cause significant damage to equipment, and even in less severe cases, the changes in the oil and gas ratio make it more difficult to ensure low amounts of discharge because the downstream equipment must adapt to the rapid changes.
These changes can also increase the need to flare, i.e., to burn off gas, which leads to increased emissions.
Therefore, mitigating slug flow and controlling production, separation, and cleaning in an optimized centralized manner can create significant value in terms of increased production and reduced emissions and discharge.
We investigate:
- mathematical modeling of the involved processes for the purpose of simulation and control
- novel methods for coordinated model-based optimizing control
- centralized and decentralized approaches for optimizing the production, separation, and cleaning of produced oil and gas.
In this project, we develop digitalization tools for simultaneously optimizing well flow and produced water quality in order to meet discharge goals and increase production.
This requires combined modelling of the near-wellbore region, the riser, the three-phase separation of the produced liquid, and de-oiling hydrocyclones (i.e., primary treatment).
Each subsystem can be operated in a decentralized manner. However, fully coordinated and centralized control can offer significant advantages in terms of:
- profits
- satisfaction of emissions and discharge limits
- mitigation of instabilities (e.g., slug flow).
We investigate both linear and nonlinear economic model predictive control (MPC) strategies, and we compare centralized and decentralized setups.
Furthermore, the MPC strategies involve:
- system identification (e.g., parameter estimation)
- state estimation
- optimal control.
The comparison will be based on both in silico simulations as well as in vivo tests on the pilot plant at AAU Esbjerg.
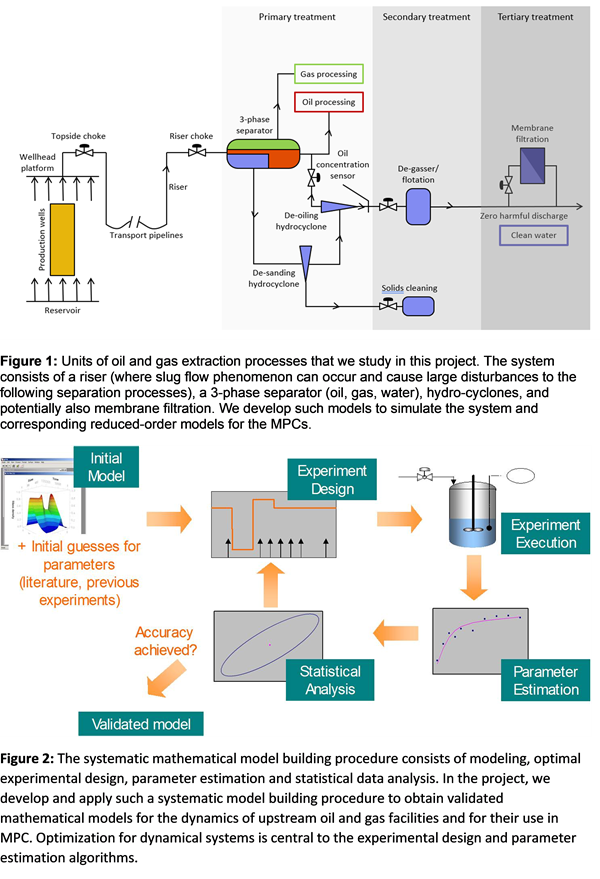
Main supervisor:
Professor John Bagterp Jørgensen, DTU Compute
Co- supervisor:
Associate Professor Zhenyu Yang, Aalborg University Esbjerg
Ali Akbar Efterkhari, DHRTC, DTU